Turning "Poop" Into Podcast Gold: An AI-Powered Solution For Repetitive Documents
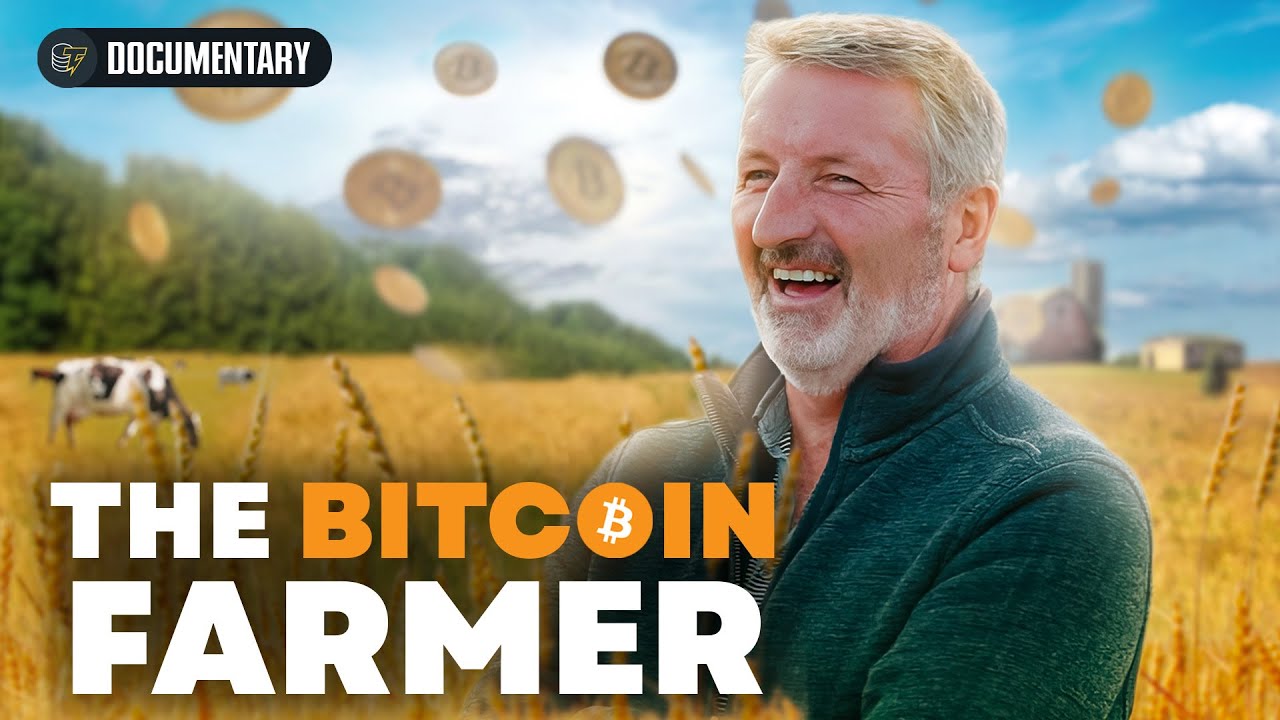
Table of Contents
Identifying and Quantifying Data Redundancy ("Poop"):
Recognizing the Problem:
Data redundancy, or "data poop" as we're playfully calling it, is a silent killer of productivity. It's more than just an organizational nuisance; it represents hidden costs that significantly impact your bottom line. The consequences of ignoring data redundancy are far-reaching. Wasted time spent searching for the correct version of a document, increased storage costs, and a higher likelihood of errors all contribute to decreased efficiency and potentially inaccurate decision-making. Imagine the frustration of dealing with conflicting information across multiple versions of the same report!
- Examples of repetitive documents: Financial reports, legal documents, medical records, marketing materials, HR forms, and countless other types of documents can suffer from significant redundancy.
- Consequences of ignoring data redundancy: Missed deadlines, inaccurate information, increased operational costs, frustrated employees, and a damaged reputation.
- The challenge of manual identification: Manually identifying repetitive content in large datasets is incredibly time-consuming, prone to errors, and frankly, impossible to scale effectively.
Measuring the Impact:
Quantifying the extent of your data redundancy is crucial for understanding its true impact. Several methods can help you measure this "data poop" problem:
- Analyzing file sizes: Large clusters of similarly sized files could indicate significant redundancy.
- Comparing document similarity: Using tools that assess text similarity can reveal near-duplicate documents. This goes beyond simple copy-pasting and detects subtle variations in wording.
- Highlighting duplicated information: Software solutions can identify and highlight specific duplicated passages within and across documents.
By measuring the impact of redundant data, you can build a strong case for implementing AI-powered solutions to tackle this issue.
AI-Powered Solutions for Data Deduplication:
Natural Language Processing (NLP):
NLP algorithms are revolutionizing how we handle data redundancy. They can identify semantically similar documents, even if they aren't exact duplicates. This is crucial because many instances of redundancy involve documents that have similar content but different wording or formatting.
- Techniques: Cosine similarity measures the angular distance between document vectors, reflecting semantic similarity. Word embeddings capture the contextual meaning of words, enabling more nuanced comparisons. Document clustering groups similar documents together based on their semantic content.
- Benefits: NLP identifies near-duplicates, surpassing the capabilities of simple comparison tools that only flag exact matches.
- Examples: NLP is used in legal tech to consolidate similar case documents, in research to identify duplicate publications, and in marketing to optimize content strategy by avoiding redundant messaging.
Machine Learning (ML) for Pattern Recognition:
ML models are particularly effective at identifying and classifying repetitive information within large and complex datasets. They can learn patterns and automatically flag potentially redundant content.
- Supervised vs. unsupervised learning: Supervised learning uses labelled data to train models to identify redundancy, while unsupervised learning can uncover hidden patterns without pre-labelled data.
- Training data requirements: The quality and quantity of training data are critical for the accuracy and effectiveness of ML models.
- Benefits: ML handles large and complex datasets efficiently, reducing the need for manual intervention and improving scalability.
Practical Applications and Case Studies:
Streamlining Workflow in Various Industries:
AI-powered data deduplication is transforming workflows across various industries:
- Example 1 (Legal): Legal firms are using AI to consolidate similar case documents, reducing research time and improving efficiency.
- Example 2 (Healthcare): Healthcare providers use AI for patient record deduplication, ensuring data accuracy and improving patient care.
- Example 3 (Finance): Financial institutions use AI to detect and prevent fraudulent transactions based on repetitive patterns in financial data.
Quantifiable Results and ROI:
The benefits of AI-driven data deduplication are significant and quantifiable:
- Reduced processing time: AI dramatically reduces the time spent identifying and managing redundant data.
- Lower operational costs: Reduced manual effort translates to significant cost savings.
- Improved data accuracy: Eliminating redundant data ensures data consistency and reliability.
Choosing the Right AI-Powered Solution:
Factors to Consider:
Choosing the right AI-powered solution requires careful consideration of several factors:
-
Scalability: The solution must be able to handle your current data volume and future growth.
-
Integration: Seamless integration with existing systems is essential for smooth implementation.
-
Data security: Protecting sensitive data is paramount; choose a solution that meets your security requirements.
-
Cost: Evaluate the cost of implementation, maintenance, and ongoing support.
-
Vendor support: Reliable vendor support is essential for troubleshooting and ongoing assistance.
-
Cloud-based vs. on-premise: Cloud-based solutions offer scalability and flexibility, while on-premise solutions provide greater control over data security.
-
Open-source vs. proprietary software: Open-source solutions offer greater flexibility and customization, but may require more technical expertise.
-
Importance of data security and privacy: Data security and privacy regulations (like GDPR) must be carefully considered when choosing a solution.
Conclusion:
Using AI to address data redundancy offers numerous benefits, transforming the overwhelming "poop" (repetitive data) into valuable insights and significant efficiency gains. By implementing AI-powered solutions, you can dramatically reduce processing time, lower operational costs, and improve data accuracy.
Ready to stop drowning in repetitive documents and start turning your "data poop" into podcast gold? Explore AI-powered solutions today and unlock the potential of efficient data management! Learn more about AI-driven data deduplication and optimize your workflow now.
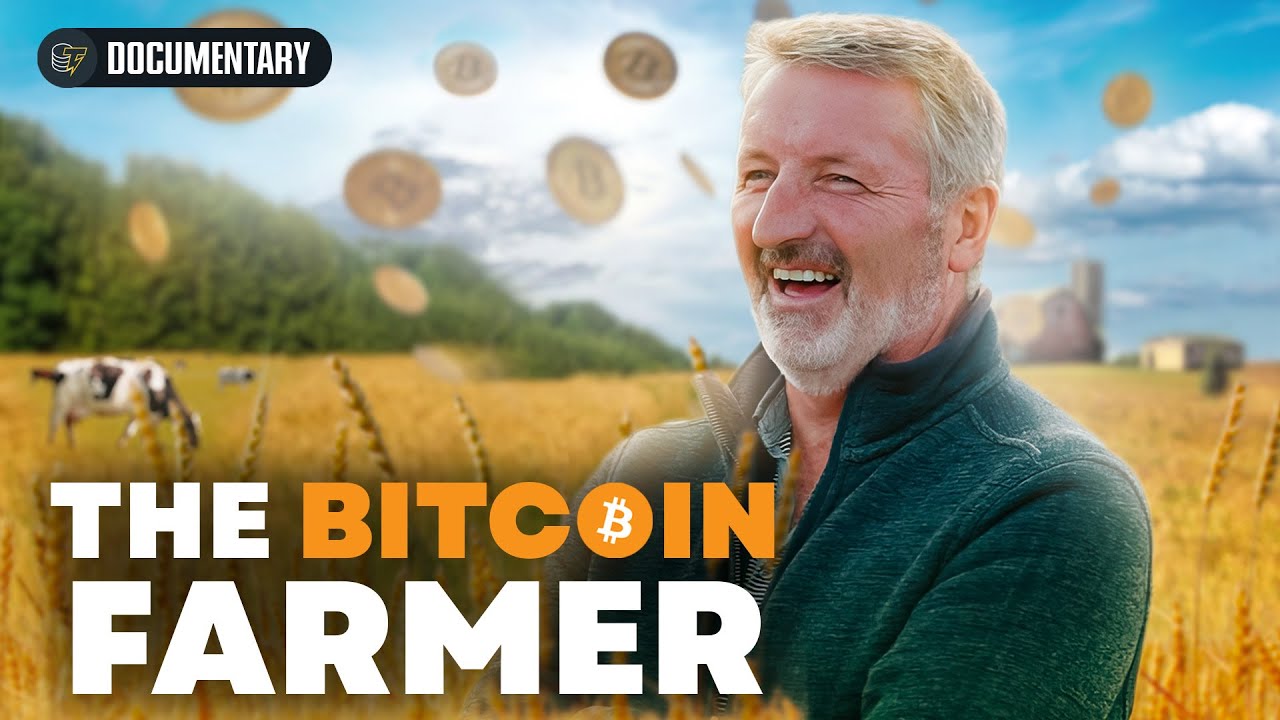
Featured Posts
-
Antlaq Fealyat Fn Abwzby Fy 19 Nwfmbr
Apr 28, 2025 -
Addressing The O Neill Absence Red Sox Roster Options For 2025
Apr 28, 2025 -
A 2000 Yankees Diary Examining Joe Torres Leadership And Andy Pettittes Shutout
Apr 28, 2025 -
Mets Opening Day Roster Prediction Early Spring Training Insights
Apr 28, 2025 -
Tylor Megill Key Factors Behind His Strong Performance For The New York Mets
Apr 28, 2025
Latest Posts
-
The Most Emotional Rocky Film According To Sylvester Stallone
May 11, 2025 -
Rockys Emotional Core Stallones Favorite Film Explored
May 11, 2025 -
Sylvester Stallones Favorite Rocky Movie The Most Emotional Entry
May 11, 2025 -
Stallones Behind The Camera Misfire A Look At His Unsuccessful Directorial Debut
May 11, 2025 -
Did Sylvester Stallone Regret Rejecting A Role In Coming Home 1978
May 11, 2025