Using AI To Digest Repetitive Scatological Documents For Podcast Production
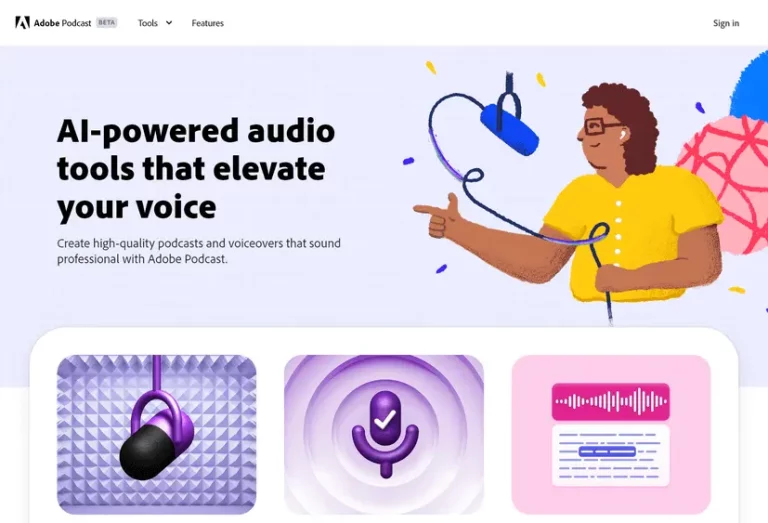
Table of Contents
Identifying the Challenges of Manual Scatological Data Processing
Manually reviewing large volumes of scatological data presents significant challenges for podcast producers. The process is not only time-consuming but also prone to human error, potentially impacting the accuracy and overall quality of the final product.
Time Consumption
Manually reviewing scatological data is incredibly time-intensive, delaying the podcast production process and impacting deadlines.
- Hours spent on manual review: Time spent manually sifting through data could be used for creative content development, research, or marketing efforts. This lost time translates directly into lost opportunity.
- Missed deadlines: The slow pace of manual data processing can lead to missed deadlines, impacting release schedules and potentially upsetting listeners and sponsors.
- Increased production costs: The substantial labor hours dedicated to manual data analysis significantly increase production costs, eating into profit margins.
Human Error
Manual data entry and analysis are inherently prone to human error, leading to inaccuracies and potentially misrepresenting information in the podcast.
- Typos and misinterpretations: Errors in transcription or interpretation can significantly alter the meaning of the original data.
- Inconsistent data formatting and analysis: Lack of standardization in data processing can lead to inconsistencies and unreliable results.
- Risk of overlooking crucial details: The sheer volume of data makes it easy to miss key details during manual review, impacting the completeness and accuracy of the podcast's narrative.
AI Solutions for Efficient Scatological Data Analysis
Fortunately, AI offers several solutions to streamline and improve the processing of scatological data for podcast production. These solutions significantly reduce the time and effort required while minimizing the risk of human error.
Automated Transcription and Summarization
AI-powered transcription services offer a revolutionary approach to handling scatological documents. They quickly and accurately transcribe audio or video recordings, even those containing sensitive or unconventional language, converting them into easily searchable text. Further, advanced AI algorithms can summarize key themes and insights, drastically reducing the manual review time.
- Reduced transcription costs and time: AI significantly reduces the cost and time associated with manual transcription, freeing up resources for other tasks.
- Improved accuracy compared to human transcription: AI transcription services boast higher accuracy rates than manual transcription, reducing the risk of errors.
- Easy integration with various podcast editing software: Many AI transcription services offer seamless integration with popular podcast editing software, streamlining the workflow.
Data Cleaning and Filtering
AI algorithms excel at cleaning and filtering irrelevant or redundant information from scatological documents. This focuses the analysis on the most pertinent details for podcast content creation, maximizing efficiency and accuracy.
- Efficient removal of unnecessary data points: AI can automatically remove irrelevant data, reducing the volume of information that needs manual review.
- Improved data quality and reliability: By eliminating extraneous information, AI ensures higher quality and more reliable data for analysis.
- Focus on critical information relevant to the podcast narrative: AI helps highlight the information most relevant to your podcast's story, saving valuable time and improving the overall quality of your content.
Sentiment Analysis and Topic Modeling
Sophisticated AI tools can perform sentiment analysis, identifying the emotional tone and key themes expressed within the scatological documents. This provides crucial insights for informing podcast discussions and content strategy.
- Understanding audience reactions and opinions: Sentiment analysis helps understand audience perceptions and opinions regarding the discussed topics.
- Identifying sensitive topics that require careful handling: AI can help identify potentially sensitive topics requiring careful consideration and nuanced discussion.
- Deeper insights into the data for richer podcast content: By uncovering hidden themes and patterns, AI helps create more insightful and engaging podcast content.
Conclusion
The use of AI to digest repetitive scatological documents for podcast production offers a significant advantage. By automating data analysis and transcription, podcast producers can significantly improve efficiency, reduce costs, and enhance accuracy. Embracing AI tools frees up valuable time for creative aspects, leading to higher-quality podcasts and increased productivity. Don't let tedious data processing hinder your workflow; explore the power of AI to revolutionize your scatological document analysis and create more impactful podcasts. Start exploring AI-powered solutions today to transform your podcasting workflow!
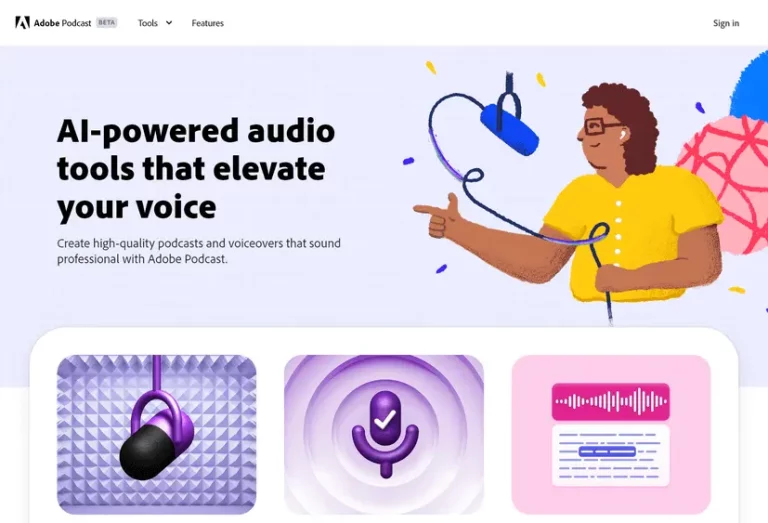
Featured Posts
-
Possible Successors To Pope Francis A Look At Potential Candidates
May 11, 2025 -
Mueller Lockas Bort Fran Bayern Intresse Fran Tva Klubbar
May 11, 2025 -
Ill House U Neal Mc Clellands Dance Track Explored Featuring Andrea Love
May 11, 2025 -
Lily Collins Sexy New Calvin Klein Campaign Photos
May 11, 2025 -
Chantal Ladesou Pourquoi Elle A Manque L Emission
May 11, 2025