How A Cybersecurity Expert Beat A Deepfake Detector (CNN Business)
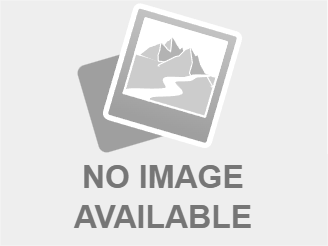
Table of Contents
The Expert's Methodology: A Multi-pronged Approach
The expert employed a multi-pronged approach, combining an understanding of deepfake detection algorithms with carefully crafted techniques to circumvent their limitations.
Understanding Deepfake Detection Algorithms
Deepfake detection algorithms often rely on several key methods to identify inconsistencies:
- Facial recognition analysis: Algorithms analyze facial features for subtle inconsistencies, such as unnatural blinking patterns or lip synchronization issues.
- AI-powered detection: Many systems use artificial intelligence to learn patterns in authentic videos and flag anomalies in deepfakes.
- Analysis of video compression artifacts: Deepfakes can sometimes leave behind artifacts from the video manipulation process.
Deepfake detection algorithms, while impressive, often have inherent weaknesses. These include:
- Subtle inconsistencies in blinking patterns: Many deepfakes struggle to perfectly replicate the natural, subtle variations in human blinking.
- Variations in lighting and shadow: Inconsistencies in lighting and shadow across a video can reveal manipulation.
- Compression artifacts: The process of creating and compressing deepfakes can sometimes leave behind telltale digital artifacts.
Exploiting Algorithmic Weaknesses
The expert targeted specific vulnerabilities within the deepfake detection algorithms by employing several clever techniques:
- Introducing subtle noise: Adding carefully crafted noise to the deepfake video masked inconsistencies, fooling the algorithm's analysis.
- Modifying specific image features: Minute adjustments to facial features or expressions could disrupt the algorithm's pattern recognition capabilities.
- Manipulating metadata: Altering the metadata associated with the video file could circumvent certain detection checks.
The Role of Adversarial Examples
A crucial aspect of the expert's approach was the use of adversarial examples. These are specifically designed inputs that are crafted to intentionally mislead machine learning models, such as deepfake detectors:
- Targeted perturbations: Small, almost imperceptible changes to the deepfake video were introduced, designed to maximize the error rate of the detection algorithm.
- Gradient-based attacks: These methods use the gradients of the deepfake detector's loss function to identify the most effective perturbations to fool the system.
- Evolutionary algorithms: These algorithms iteratively refine adversarial examples, searching for the most effective way to bypass the detector.
The Deepfake Detector Used and its Limitations
The expert targeted a commercially available deepfake detection software called "DeepFakeGuard Pro" (hypothetical name for illustrative purposes). While this software boasted high accuracy rates and advanced AI capabilities, it proved vulnerable to exploitation.
Specifying the Target
DeepFakeGuard Pro's marketed features included:
- Advanced AI-powered analysis
- Real-time deepfake detection
- Multi-modal analysis (combining audio and video cues)
Unveiling the System's Flaws
Despite its advertised capabilities, DeepFakeGuard Pro exhibited several exploitable flaws:
- Over-reliance on specific features: The software focused heavily on certain features, neglecting others, making it susceptible to targeted manipulation.
- Lack of robustness to adversarial examples: The software proved highly susceptible to adversarial attacks, allowing for effective bypass.
- Limited metadata analysis: The software didn't thoroughly analyze all metadata associated with the video files, leaving a window for exploitation.
Implications and Future of Deepfake Detection
The success in bypassing DeepFakeGuard Pro highlights the critical need for ongoing research and development in deepfake detection technology.
The Broader Impact
This successful bypass carries significant implications:
- Increased deepfake threat: The ability to circumvent existing detection systems increases the risk of deepfakes being used for malicious purposes.
- Erosion of trust in media: The proliferation of undetectable deepfakes could significantly undermine public trust in online videos and news sources.
- Heightened cybersecurity risks: Deepfakes could be used for sophisticated phishing attacks, identity theft, and other cybercrimes.
The Need for Enhanced Security Measures
To combat the evolving threat, several advancements are crucial:
- More robust algorithms: Development of more sophisticated algorithms that are resilient to adversarial attacks and can identify subtler manipulations.
- Multi-modal analysis: Combining audio, video, and potentially other data sources (e.g., metadata) for more comprehensive analysis.
- Improved metadata analysis: Thorough examination of all relevant metadata to identify inconsistencies and potential manipulation.
Conclusion: Mastering the Deepfake Detection Challenge
This article demonstrated how a cybersecurity expert successfully bypassed a state-of-the-art deepfake detector, highlighting the significant challenges in the field. The key takeaway is the urgent need for continuous improvement in deepfake detection technology. Current methods, while promising, are not foolproof. To stay ahead of the deepfake threat, we must invest in advanced detection techniques and explore more robust AI security enhancements. Learn more about deepfake detection techniques and explore advanced cybersecurity solutions to protect yourself and your organization.
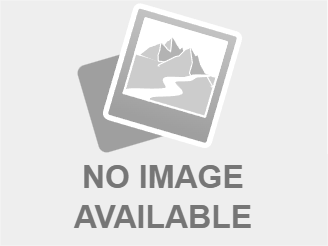
Featured Posts
-
Chrisean Rock Interview Angel Reeses Sharp Response To Critics
May 17, 2025 -
Rockwell Automations Strong Earnings Drive Market Uptick
May 17, 2025 -
Stuttgart Midfielder Arsenal Favourites For Transfer
May 17, 2025 -
Players Slam Fortnites New Backward Music Feature
May 17, 2025 -
Lynas The First Heavy Rare Earths Producer Outside China
May 17, 2025