Quantum Computing's Role In AI-Enhanced Drug Discovery: A D-Wave (QBTS) Perspective
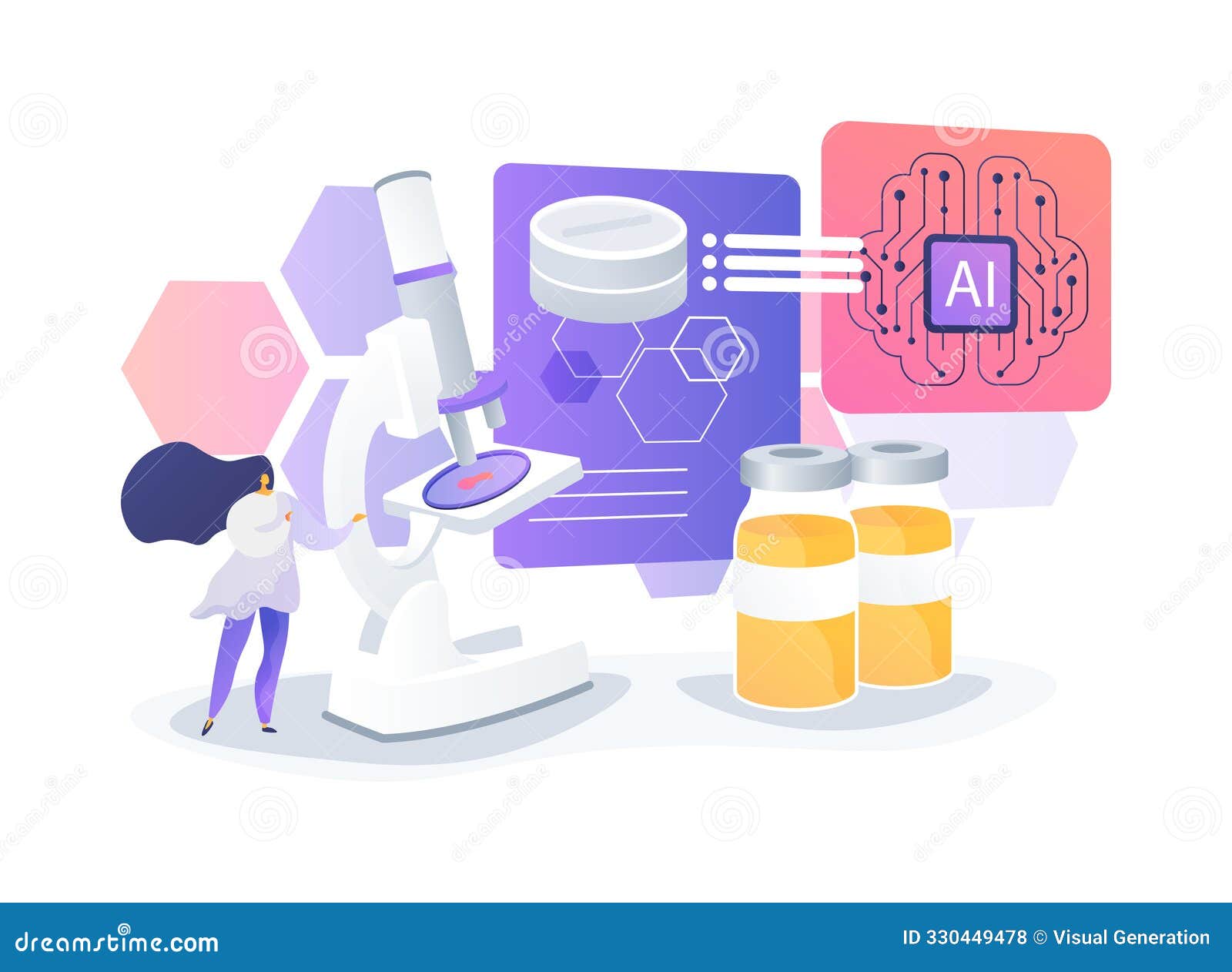
Table of Contents
Accelerating Molecular Simulations with Quantum Annealing
The Computational Bottleneck in Drug Discovery
Traditional drug discovery relies heavily on molecular simulations to understand the interactions between drug molecules and their biological targets. These simulations are incredibly complex, involving massive datasets and intricate calculations far exceeding the capabilities of even the most powerful classical computers. This computational bottleneck significantly slows down the drug discovery process, limiting the exploration of potential drug candidates and hindering the optimization of their properties. Classical algorithms struggle with the exponential complexity of many drug discovery problems.
Quantum Annealing's Advantage
D-Wave's quantum annealers, leveraging their unique Quantum-Based Technology Solutions (QBTS), offer a powerful alternative. Quantum annealing excels at solving optimization problems, a core challenge in drug discovery. These problems include:
- Protein folding: Predicting the three-dimensional structure of proteins is crucial for understanding their function and designing drugs that interact with them. Quantum annealing can significantly speed up the search for the lowest energy state, representing the most stable protein conformation.
- Ligand docking: This process involves identifying how well a potential drug molecule (ligand) fits into a protein's binding site. Quantum annealing can efficiently explore a vast chemical space to identify optimal ligand candidates.
- Lead optimization: Once a promising drug candidate is identified, its properties need to be optimized for efficacy and safety. Quantum annealing can help refine the molecular structure, improving its binding affinity and reducing potential side effects.
Specific applications in drug design include:
- Identifying novel drug candidates with improved efficacy.
- Optimizing the pharmacokinetic and pharmacodynamic properties of drug candidates.
- Predicting drug-drug interactions and potential toxicity.
Enhancing AI Algorithms with Quantum Data
AI's Role in Drug Discovery
Artificial intelligence (AI), particularly machine learning, is transforming drug discovery. AI algorithms are used in various stages:
- Target identification: Identifying promising biological targets for drug intervention.
- Lead discovery: Screening vast libraries of compounds to identify potential drug candidates.
- Lead optimization: Refining the properties of promising candidates.
- Toxicity prediction: Assessing the potential safety risks of drug candidates.
Quantum Data Enrichment
Quantum computers can generate entirely new datasets or significantly enhance existing ones. This "quantum data enrichment" fuels more robust AI models:
- Quantum machine learning (QML) algorithms can analyze complex molecular interactions more effectively than classical algorithms, leading to more accurate predictions.
- Quantum computers can provide insights into molecular properties that are inaccessible to classical methods, enriching the training data for AI models.
- This enriched data leads to improved prediction accuracy for drug efficacy and toxicity, ultimately reducing the time and resources spent on pre-clinical and clinical trials.
D-Wave's Contribution and the QBTS Advantage
D-Wave's Quantum Annealing Technology
D-Wave's quantum annealers utilize a unique approach to quantum computing, focusing on solving specific optimization problems. Their Quantum-Based Technology Solutions (QBTS) provide a practical pathway for integrating quantum computing into real-world applications, including drug discovery. This technology allows for the exploration of a much larger solution space compared to classical methods, accelerating the discovery and optimization of novel drug molecules.
Case Studies and Real-World Applications
D-Wave has been actively involved in several collaborations aimed at applying its quantum technology to drug discovery. While specific details of ongoing projects may be confidential, the potential impact is significant:
- Partnerships with pharmaceutical companies and research institutions are actively exploring the application of D-Wave's quantum annealers for various drug discovery tasks.
- Successful case studies showcasing the effectiveness of D-Wave's technology in solving specific drug design problems are emerging, demonstrating the potential to accelerate the entire drug development pipeline.
Addressing Challenges and Future Outlook
Current Limitations
Despite the significant potential, current quantum computing technology faces certain limitations:
- Qubit coherence and scalability remain challenges. Current quantum annealers have a limited number of qubits compared to the vast computational needs of some drug discovery problems.
- The development of efficient quantum algorithms specifically tailored for drug discovery is an ongoing area of research.
Future Potential
The future holds immense promise for quantum computing in drug discovery:
- Advancements in qubit technology will lead to more powerful and scalable quantum computers.
- The development of hybrid quantum-classical algorithms will combine the strengths of both classical and quantum computing, addressing the limitations of current technology. These hybrid approaches will likely become increasingly important in the near future.
- Improved understanding of quantum phenomena related to molecular interactions will lead to the design of more effective quantum algorithms.
Conclusion: The Quantum Leap in Drug Discovery
Quantum computing, specifically D-Wave's quantum annealing technology and its QBTS approach, has the potential to dramatically accelerate AI-enhanced drug discovery. By tackling the computational bottlenecks in molecular simulations and enriching AI algorithms with novel quantum data, this technology promises to reduce the time and cost associated with bringing life-saving drugs to market. The advancements in qubit coherence and scalability, along with the development of increasingly sophisticated quantum algorithms, will further unlock the immense potential of Quantum Computing's Role in AI-Enhanced Drug Discovery. To learn more about D-Wave's quantum computing solutions and their applications in AI-driven drug discovery, explore their resources and consider further research on the exciting intersection of quantum computing and pharmaceutical innovation using QBTS.
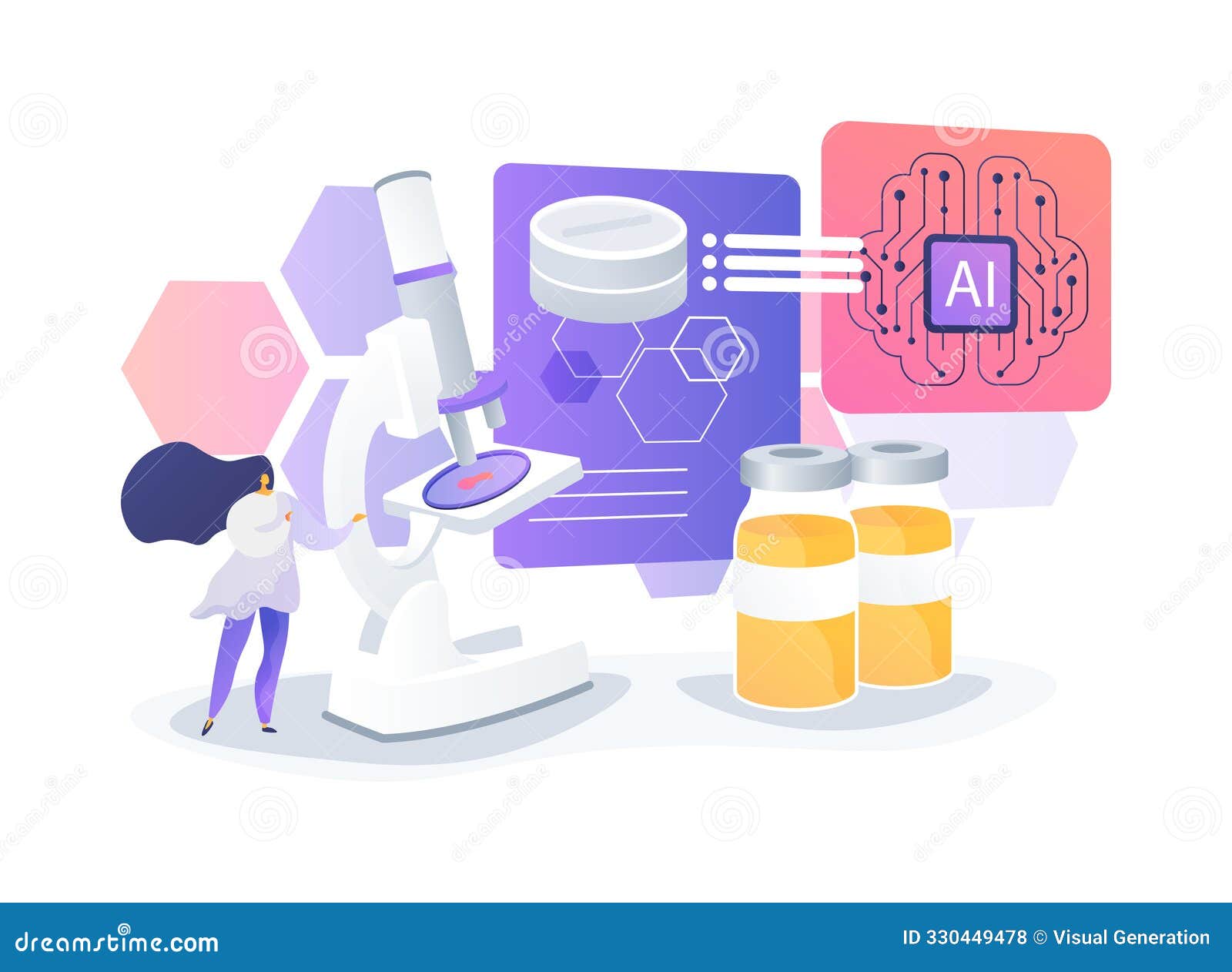
Featured Posts
-
Examining The Plot Of Agatha Christies Towards Zero Episode 1 The Absence Of Homicide
May 20, 2025 -
Manaus Festival Da Cunha Com Maiara E Maraisa Confirmado Por Isabelle Nogueira
May 20, 2025 -
Ajax Fenerbahce Den Yildiz Oyuncuyu Transfer Etti
May 20, 2025 -
The Fight For American Factory Jobs Who Will Fill Them
May 20, 2025 -
Tadic Incident Fenerbahce Kondigt Strenge Actie Tegen Ajax Aan
May 20, 2025